Matthew Olckers
Postdoctoral Researcher
Macquarie University
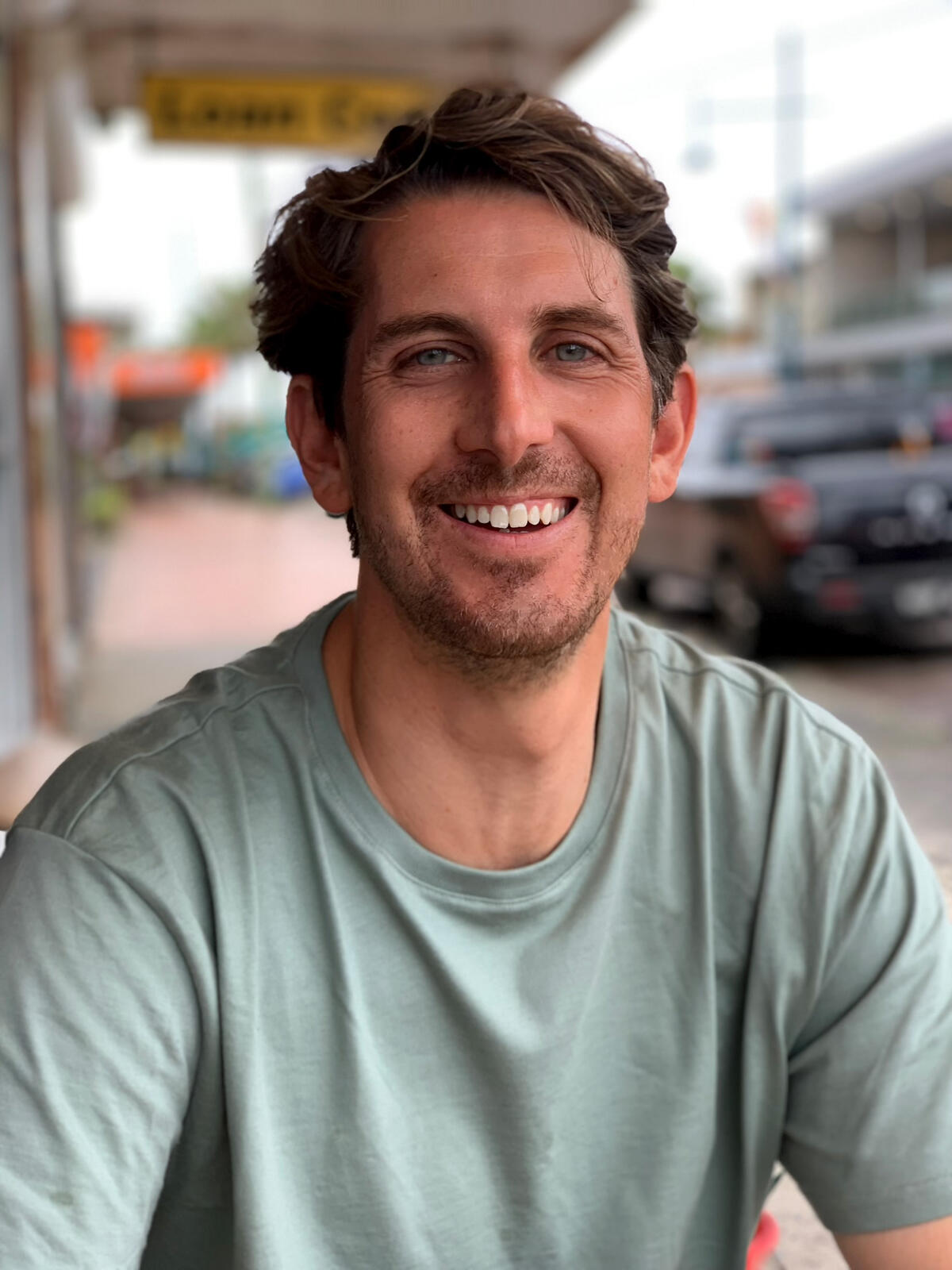
Hi, I'm Matthew Olckers. Welcome to my research website.
I work as a research fellow at Macquarie University, based at the e61 Institute.My research interests include social networks, development economics, and household finance. I am particularly interested in the intersection of economics and computer science, such as using mechanism design in practical applications or using alternative data sources to answer questions about poverty and development.I serve on the leadership of the Equity and Access in Algorithms, Mechanisms, and Optimization (EAAMO) initiative and I am an affiliate of SoDa Labs.Outside of my research, I love spending time with my family, surfing, helping out at church, and seeing graffiti on trains. I compiled a book about graffiti in Cape Town, South Africa, which subsequently evolved into a documentary.
Current Research
I use tools from economics and computer science to study a range of topics.I am working on projects in the following areas:

Rejection experiences
Rejected applicants for job and university applicants usually receive generic feedback—if they receive any feedback at all. Can techniques from AI provide personalized explanations? What are the barriers to provide personalized explanations?

Peer information
Through social interaction, we learn about our peers. For example, we may learn which of our peers is in need of financial aid. How can a social planner use peer information to target aid to the most needy individuals? How can the planner prevent manipulation of a targeting mechanism that relies on peer information?

Household finance
Household finance focusses on how we manage our money. How much do we save for retirement? How do we use credit? Do we take risks? Which types of financial products do we use?
Working Papers
What Type of Explanation Do Rejected Job Applicants Want? Implications for Explainable AI
with Alicia Vidler and Toby WalshRejected job applicants seldom receive explanations from employers. Techniques from Explainable AI (XAI) could provide explanations at scale. Although XAI researchers have developed many different types of explanations, we know little about the type of explanations job applicants want. We use a survey of recent job applicants to fill this gap. Our survey generates three main insights. First, the current norm of, at most, generic feedback frustrates applicants. Second, applicants feel the employer has an obligation to provide an explanation. Third, job applicants want to know why they were unsuccessful and how to improve.
Incentives to Offer Algorithmic Recourse
with Toby WalshDue to the importance of artificial intelligence (AI) in a variety of high-stakes decisions, such as loan approval, job hiring, and criminal bail, researchers in Explainable AI (XAI) have developed algorithms to provide users with recourse for an unfavorable outcome. We analyze the incentives for a decision-maker to offer recourse to a set of applicants. Does the decision-maker have the incentive to offer recourse to all rejected applicants? We show that the decision-maker only offers recourse to all applicants in extreme cases, such as when the recourse process is impossible to manipulate. Some applicants may be worse off when the decision-maker can offer recourse.
Gamblers Learn from Experience
with Joshua BlumenstockMobile phone-based sports betting has exploded in popularity in many African countries. Commentators worry that low-ability gamblers will not learn from experience, and may rely on debt to gamble. Using data on financial transactions for over 50 000 Kenyan smartphone users, we find that gamblers do learn from experience. Gamblers are less likely to bet following poor results and more likely to bet following good results. The reaction to positive and negative feedback is of equal magnitude and is consistent with a model of Bayesian updating. Using an instrumental variables strategy, we find no evidence that increased gambling leads to increased debt.
In Progress
Publications
Journals
2024
Manipulation and Peer Mechanisms: A Survey
Matthew Olckers, Toby Walsh
Artificial Intelligence, 336 (104196)
2022
Friend-Based Ranking
Francis Bloch, Matthew Olckers
American Economic Journal: Microeconomics, 14 (2): 176-214
2021
On Track for Retirement?
Matthew Olckers
Journal of Economic Behavior & Organization, 190: 76-88
2020
Four Levers of Redistribution: The Impact of Tax and Transfer Systems on Inequality Reduction
Elvire Guillaud, Matthew Olckers, Michaël Zemmour
Review of Income and Wealth, 66 (2): 444-466
Conference Proceedings
2024
Bridging Research and Practice Through Conversation: Reflecting on Our Experience
Mayra Russo, Mackenzie Jorgensen, Kristen M. Scott, Wendy Xu, Di H. Nguyen, Jessie Finocchiaro, Matthew Olckers
Proceedings of the 4th ACM Conference on Equity and Access in Algorithms, Mechanisms, and Optimization, (in press)
2021
Friend-Based Ranking in Practice
Francis Bloch, Matthew Olckers
AEA Papers and Proceedings, 111: 567-71